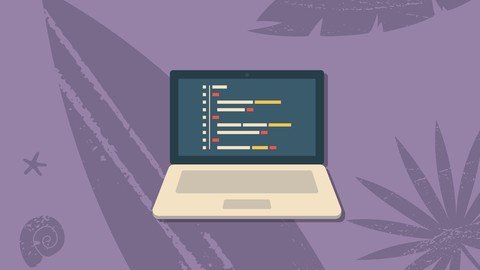
Last updated 9/2017
MP4 | Video: h264, 1280x720 | Audio: AAC, 44.1 KHz
Language: English | Size: 443.96 MB | Duration: 4h 4m
Tackle programming problems and explore model-based and model-free learning algorithms for reward-based learning in R
What you'll learn
Get to know the nuts and bolts of writing R code in RStudio
Get a tour of the most important data structures in R
Execute environment and Q-Learning functions with R
Learn episode and state-action functions in R
Master Q-Learning with Greedy Selection examples in R
Explore simulated annealing changed discount factor through examples in R
Requirements
Basic programming knowledge
Basic knowledge of math and statistics would be beneficial
Description
R is a high-level statistical language and is widely used among statisticians and data miners to develop statistical applications. If you want to learn reward-based learning with R, then you should surely go for this Learning Path.
Packt's Video Learning Paths are a series of individual video products put together in a logical and stepwise manner such that each video builds on the skills learned in the video before it.
The highlights of this Learning Path are
Tour of the most important data structures in RImplement applications for model-based and model-free RL
Beginning with the basics of R programming, this Learning Path provides step-by-step resources and time-saving methods to help you solve programming problems efficiently. You will be able to boost your productivity with the most popular R packages and data structures such as matrices, lists, and factors. You will be able to tackle issues with data input/output and will learn to work with strings and dates.
Moving ahead, you will know the differences in model-free and model-based approaches to reinforcement learning. This Learning Path discusses the characteristics, advantages and disadvantages, and typical examples of model-free and model-based approaches.You will learn Monte Carlo approach, Q-Learning approach, SARSA approach, and many more. Finally, you will take a look at model-free simulated annealing and more Q-Learning algorithms.
By the end of this Learning Path, you will be able to build actions, rewards, and punishments through these models in R for reinforcement learning.
About the Author
For this course, we have the best works of this esteemed authors
Dr David Wilkins is a biologist with nearly a decade of experience writing R for research applications, particularly high-throughput analysis of genetic data. He has also developed a number of open source R packages.Dr. Geoffrey Hubona held a full-time tenure-track, tenured, assistant and associate professor faculty positions at three major state universities in the Eastern United States from 1993-2010. In these positions, he taught dozens of various statistics, business information systems, and computer science courses to undergraduate, master's and Ph.D. students. Dr. Hubona earned a Ph.D. in Business Administration (Information Systems and Computer Science) from the University of South Florida (USF) in Tampa, FL (1993); an MA in Economics (1990), also from USF; an MBA in Finance (1979) from George Mason University in Fairfax, VA; and a BA in Psychology (1972) from the University of Virginia in Charlottesville, VA.
Overview
Section 1: Learn R programming
Lecture 1 The Course Overview
Lecture 2 Setting Up RStudio
Lecture 3 Writing, Running, and Saving R Scripts
Lecture 4 Exploring Numbers and Arithmetic Operators
Lecture 5 Working with Variables and Vectors
Lecture 6 Using Functions and Reading Function Documentation
Lecture 7 Exploring Vectors in Depth and Understanding Data Types
Lecture 8 Working with Matrices and Arrays
Lecture 9 Discovering Lists
Lecture 10 Discovering Data Frames
Lecture 11 Exploring Factors
Lecture 12 Reading Data from a File
Lecture 13 Subsetting Data Frames
Lecture 14 Statistical Summaries of Data
Lecture 15 Statistical Tests on Data
Lecture 16 Manipulating Data
Lecture 17 Writing Data to File
Section 2: Discover Algorithms for Reward-Based Learning in R
Lecture 18 The Course Overview
Lecture 19 R Example – Building Model-Free Environment
Lecture 20 R Example – Finding Model-Free Policy
Lecture 21 R Example – Finding Model-Free Policy (Continued)
Lecture 22 R Example – Validating Model-Free Policy
Lecture 23 Policy Evaluation and Iteration
Lecture 24 R Example – Moving a Pawn with Changed Parameters
Lecture 25 Discount Factor and Policy Improvement
Lecture 26 Monte Carlo Methods
Lecture 27 Environment and Q-Learning Functions with R
Lecture 28 Learning Episode and State-Action Functions in R
Lecture 29 State-Action-Reward-State-Action (SARSA)
Lecture 30 Simulated Annealing – An Alternative to Q-Learning
Lecture 31 Q-Learning with a Discount Factor
Lecture 32 Visual Q-Learning Examples
This Learning Path is for programmers, data analyst, or data science enthusiasts who want to learn reward-based learning with R. No prior R knowledge is required as the Learning Path covers the fundamental concepts of R.
Homepage
https://www.udemy.com/course/learning-path-r-reward-based-learning-with-r/
Download From 1DL
https://1dl.net/lu1poyp5dy10/gdzbq.Learning.Path.R.RewardBased.Learning.With.R.rar

https://rapidgator.net/file/6ca30dc827c169ed058b44132aaa802a/gdzbq.Learning.Path.R.RewardBased.Learning.With.R.rar.html

https://uploadgig.com/file/download/d4fF858cBee8BF3c/gdzbq.Learning.Path.R.RewardBased.Learning.With.R.rar

https://freshwap.cc/view/D733F450BC1D9A0/gdzbq.Learning.Path.R.RewardBased.Learning.With.R.rar
Views: 0
Comments (0)
free Learning Path R Reward-Based Learning With R, Downloads Learning Path R Reward-Based Learning With R, RapidShare Learning Path R Reward-Based Learning With R, Megaupload Learning Path R Reward-Based Learning With R, Mediafire Learning Path R Reward-Based Learning With R, DepositFiles Learning Path R Reward-Based Learning With R, HotFile Learning Path R Reward-Based Learning With R, Uploading Learning Path R Reward-Based Learning With R, Easy-Share Learning Path R Reward-Based Learning With R, FileFactory Learning Path R Reward-Based Learning With R, Vip-File Learning Path R Reward-Based Learning With R, Shared Learning Path R Reward-Based Learning With R, Please feel free to post your Learning Path R Reward-Based Learning With R Download, Movie, Game, Software, Mp3, video, subtitle, sample, torrent, NFO, Crack, uploaded, putlocker, Rapidgator, mediafire, Netload, Zippyshare, Extabit, 4shared, Serial, keygen, Watch online, requirements or whatever-related comments here.
Related Downloads :